Nvidia TAO Toolkit and Advex Synthetic Data Accelerates Machine Vision Automation
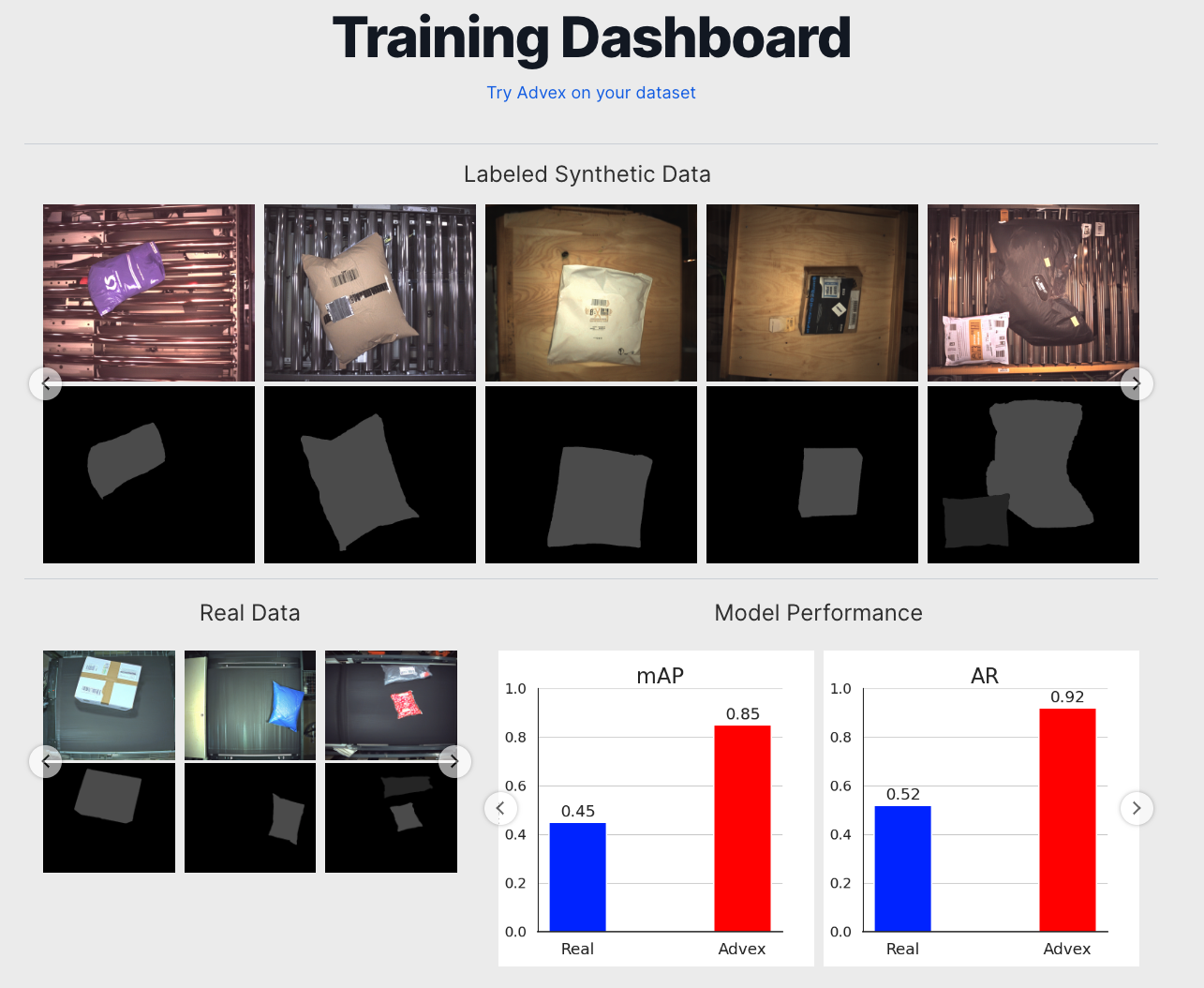
The industrial sector faces a growing demand for AI-powered vision automations in defect inspection. However, the automation is often hindered by two critical bottlenecks: time-expensive and labor-intensive creation of Machine Learning models, and insufficient defective data to train the models.
Traditionally, data scientists and AI experts must come together to create an effective and well optimized model that aligns with their intended use case. This tedious process can take months and sometimes years to complete and is resource consuming. Machine vision companies like Cognex and Keyence have made Deep Learning accessible to Automation Engineers. However, in manufacturing environments, defects are typically rare occurrences that might happen as infrequently as just a few times a year. This makes it difficult to collect and label comprehensive datasets that accurately capture every unique defect occurrence. However, training a robust computer vision system capable of accurately detecting defects in various shapes, sizes, orientations, positions, and lighting conditions requires hundreds, if not thousands, of diverse images.
To address the issues of tedious model creation and data scarcity, developers can now use NVIDIA TAO toolkit, and Advex AI’s Synthetic Data Generation Platform. TAO Model fine-tuning can take anywhere between 15 minutes to 18 hours depending on the dataset size. Advex’s platform can generate up to 5000 images in about 6 hours. These two expeditious solutions for Vision Defect Inspection can be exploited to fast-forward the industrial sector.
This post shows how by leveraging Advex's Synthetic Data Generation Platform in conjunction with NVIDIA's TAO Toolkit, manufacturers can now rapidly accelerate inspection processes across various industries, including Food & Beverage processing and packaging, PCB manufacturing, and Surface Defect detection.
Platforms for Vision Industrial Defect Inspection
NVIDIA TAO toolkit is an open-source toolkit that simplifies the process of training and optimizing models by leveraging the power of transfer learning. By using the TAO toolkit, developers can customize any of the available pre-trained models to create industry-ready models for Vision Defect Inspection in a short amount of time.
.png)
Advex AI, an NVIDIA Inception program member, led by AI researchers and Industrial Automation experts, has developed a Generative AI-powered Synthetic Data Generation Platform bringing an innovative solution to the challenge of building comprehensive datasets for Machine Learning. This enables leading manufacturers and Machine Vision providers to achieve production-level accuracy in a fraction of the time traditionally required.
Rooted in Generative Diffusion models, Advex’s platform not only accelerates initial deployment but also helps maintain high performance despite inevitable changes in environmental conditions. As Pedro Pachuca, Advex CEO, puts it: “We believe industrials are the backbone of humanity. For us, it’s about improving the lives of billions by increasing industrial automation 1000-fold. This means automating repetitive tasks like inspection and upskilling employees to do more meaningful work.”
Advex's Synthetic Data Performance on TAO models for Industrial Defect Inspection
Using randomly selected samples from 3 publicly available defective datasets of Fruits and Vegetables, PCBs, and Metal Surface, Advex’s platform was used to generate Synthetic Data.
Advex's synthetic data generation process is both efficient and scalable. Every data-generation iteration goes through the following four and occasionally five steps:
1. Data Ingestion: We upload our initial dataset of at least 10 labeled images to the Advex platform.
2. Variation Analysis: From this initial dataset, the Advex Platform comprehends the possible variations within the dataset. For instance, if the uploaded images depict metal parts with multiple defect types under various lighting conditions, Advex will generate synthetic images that reflect these variations.
3. Data Generation: Utilizing advanced diffusion models, Advex produces synthetic images that maintain the characteristics of the original dataset while introducing controlled variations in defect size, shape, position, and environmental factors.
4. Automatic Labeling: All synthetic images are automatically labeled by the Advex Platform, supporting classification, segmentation, bounding box, and key point tasks.
5. Advanced Guidance: For certain scenarios, specific variations are anticipated but not present in the initial dataset. Advex's Advanced Guidance feature was used to incorporate domain expertise. For example, if a new color variant of a part is expected in production, Advanced Guidance can generate synthetic images of parts in the specified color.
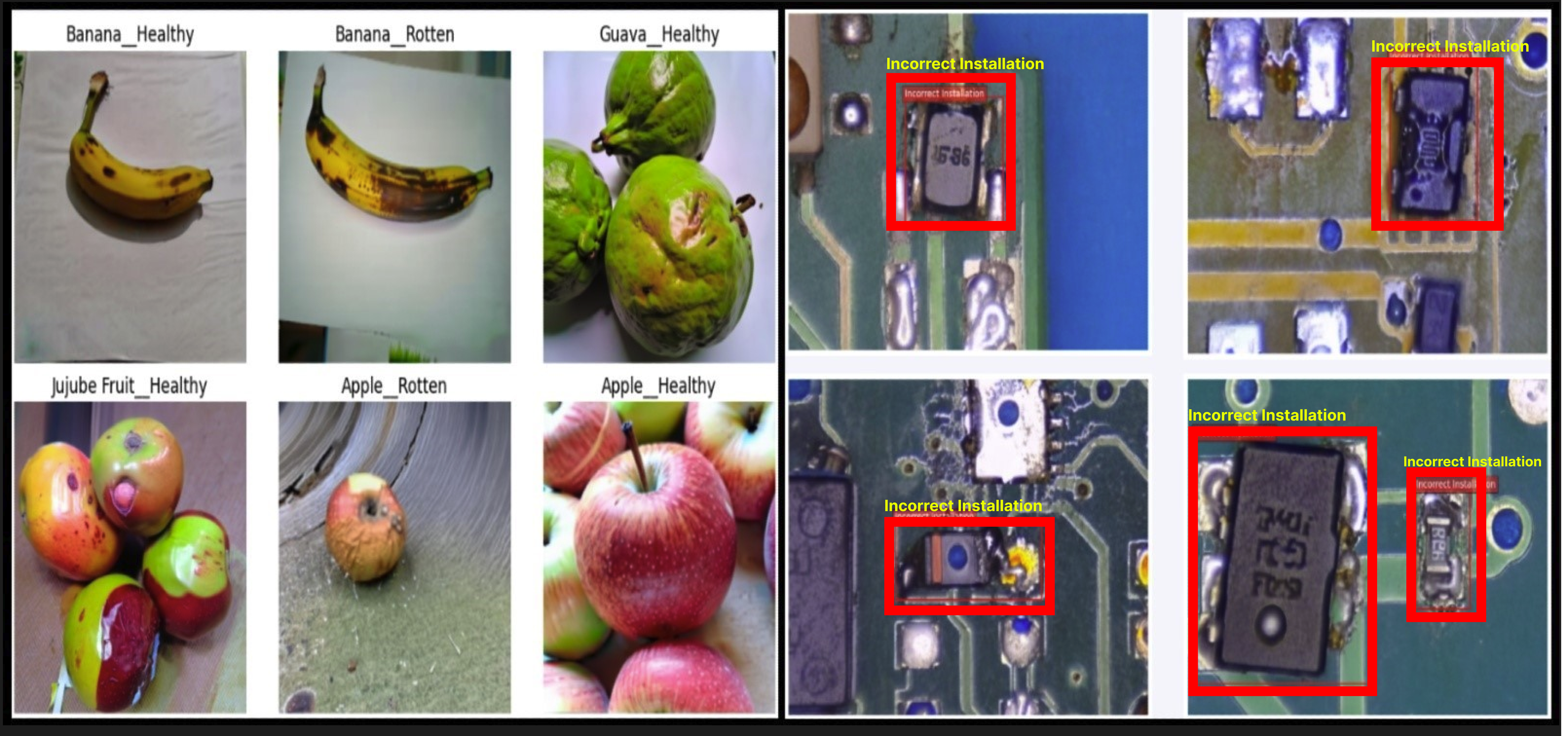
Next, the Advex generated datasets were tested on TAO’s models. To understand its usability and reflect real-world scenarios, the models were tested on both real and real + synthetic data. Then the performance on both datasets were compared.
TAO’s DINO, D-DETR, and Image Classification PyT models were used to compare the datasets. The PCB dataset was used on DINO, the Metal Surface on D-DETR, and the Fruits Dataset on Image Classification PyT. While evaluating the models, the mAP doubled on the PCB dataset when using synthetic data and tripled on the Metal dataset. Additionally, an increase of 45% of the F1 score on the Fruits Dataset was observed when the model was tested on Synthetic data.
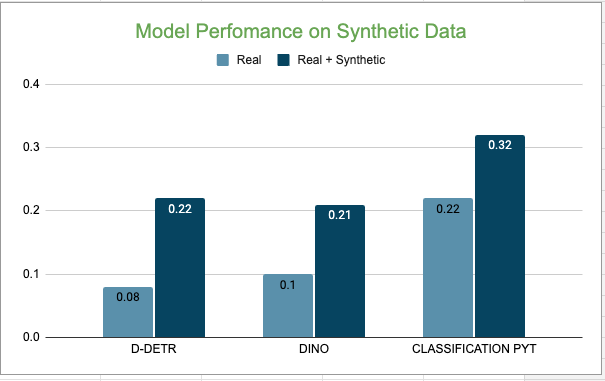
The improvement in model performance was due to multiple reasons including the fundamental differences in the models and their backbones and the differences in datasets. Ultimately, however, an increase in performance is always guaranteed regardless of the model or dataset type, emphasizing the usefulness of synthetic data especially in environments that make it hard to gather and label real data in a reasonable amount of time.
Summary
In an industry where high accuracy is fundamental, automation of repetitive tasks like defect inspection is necessary. However, lack of enough data makes this a challenging task. With Advex’s Synthetic Data and NVIDIA’s pre-trained models the issue couldn’t be more simplified. These two presents a fast and efficient solution to Vision Inspection.
“The biggest assumption we make in deep learning today is that your data is fundamentally I.I.D (independent and identically distributed) which implies that every sample is equally important. This couldn’t be further from the truth. In nature, there are samples that are clearly more valuable than others. Being able to effectively identify valuable pieces of data and create more of it is the holy grail of AI. And that’s what the Advex platform is designed to do.” - Qasim Wani, CTO
To stay competitive and ensure the highest standards of quality control, manufacturers should leverage the innovative capabilities of Advex AI’s Synthetic Data Generation Platform and NVIDIA's TAO Toolkit. By doing so, they can automate repetitive inspection tasks, enhance model accuracy, and ultimately improve productivity. Embrace this cutting-edge technology to transform your defect inspection processes and elevate your manufacturing operations to new heights.
Advex is hiring for several roles within research and engineering. If you enjoyed reading this article, consider joining the team. Reach out to qasim@advexai.com for any questions.
See the Advex Platform in action
Get Started
- Book a call with Advex on our Contact Us page.
- Learn more about NVIDIA TAO Toolkit from NVIDIA Docs and get started resources